Accelerated Materials Product Optimization with AI-Guided Experimental Design
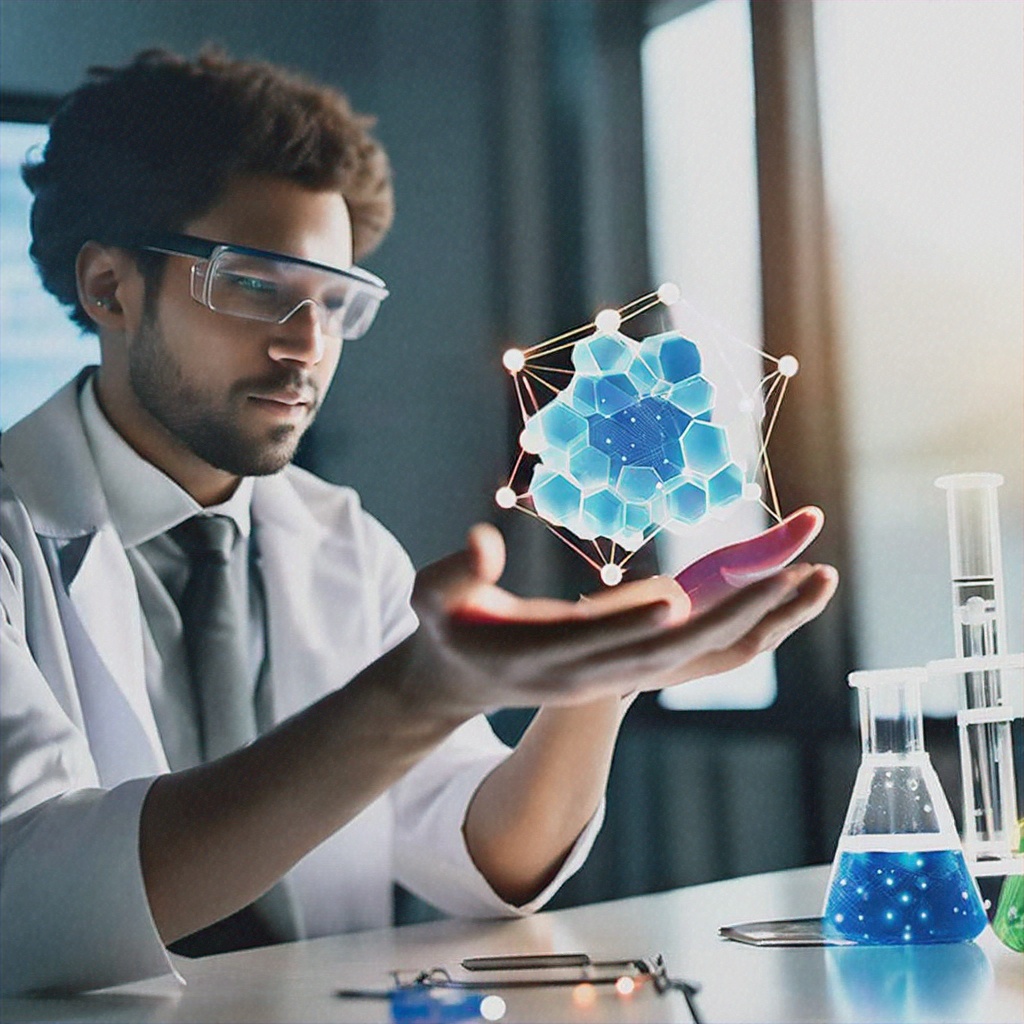

Materials research faces major challenges due to the vast number of composition and process combinations and the high costs and time required for experiments. These challenges are further complicated by the need to optimize multiple, often conflicting material properties. The MaterialsZone platform addresses these issues by combining efficient experiment documentation with advanced Machine Learning, reducing experimental iterations by over 70%.
Problem
Materials R&D is complicated by the endless combinations of materials and processes, along with expensive and time-consuming experiments. This complexity makes it hard for researchers to explore and optimize the multi-dimensional material parameter space and for managers to deliver products on schedule. The challenge is further heightened by the need to optimize several material properties simultaneously, which can sometimes be inversely related, like process cost versus product quality.

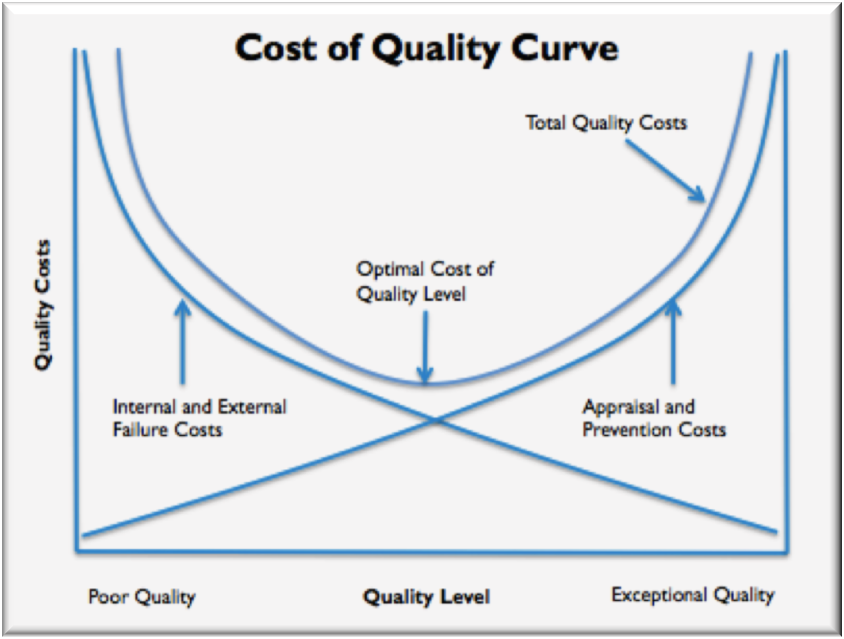
Solution
MaterialsZone’s platform helps overcome these challenges through a combination of efficient experiment documentation and advanced Machine Learning. This approach enables researchers to explore and optimize the multi-dimensional, interdependent parameter space more effectively.
Efficient Experiment Documentation
MaterialsZone’s Materials Knowledge Center allows organizations to document their experiments in a standardized, machine-learning-ready format. The Collaboration Hub enables teams to plan, execute, and document experiments on a centralized platform, ensuring transparency and collaboration.
Predictive Co-Pilot for Experimental Design
MaterialsZone’s Predictive Co-Pilot helps researchers define their problem space, constraints, and targets to guide exploration. It leverages existing data from the Materials Knowledge Center to train Machine Learning models, suggesting new experiments to optimize the parameter space. This iterative process, known as the "Active Learning Cycle," enables better results with fewer experimental iterations.

Results
The Active Learning Cycle can be implemented with minimal initial data and achieves significantly better outcomes with fewer experiments than traditional methods.

<70% fewer experimental iterations compared to traditional experimental design methods.